Emanuele Rossi
Graph Machine Learning @ Imperial College London
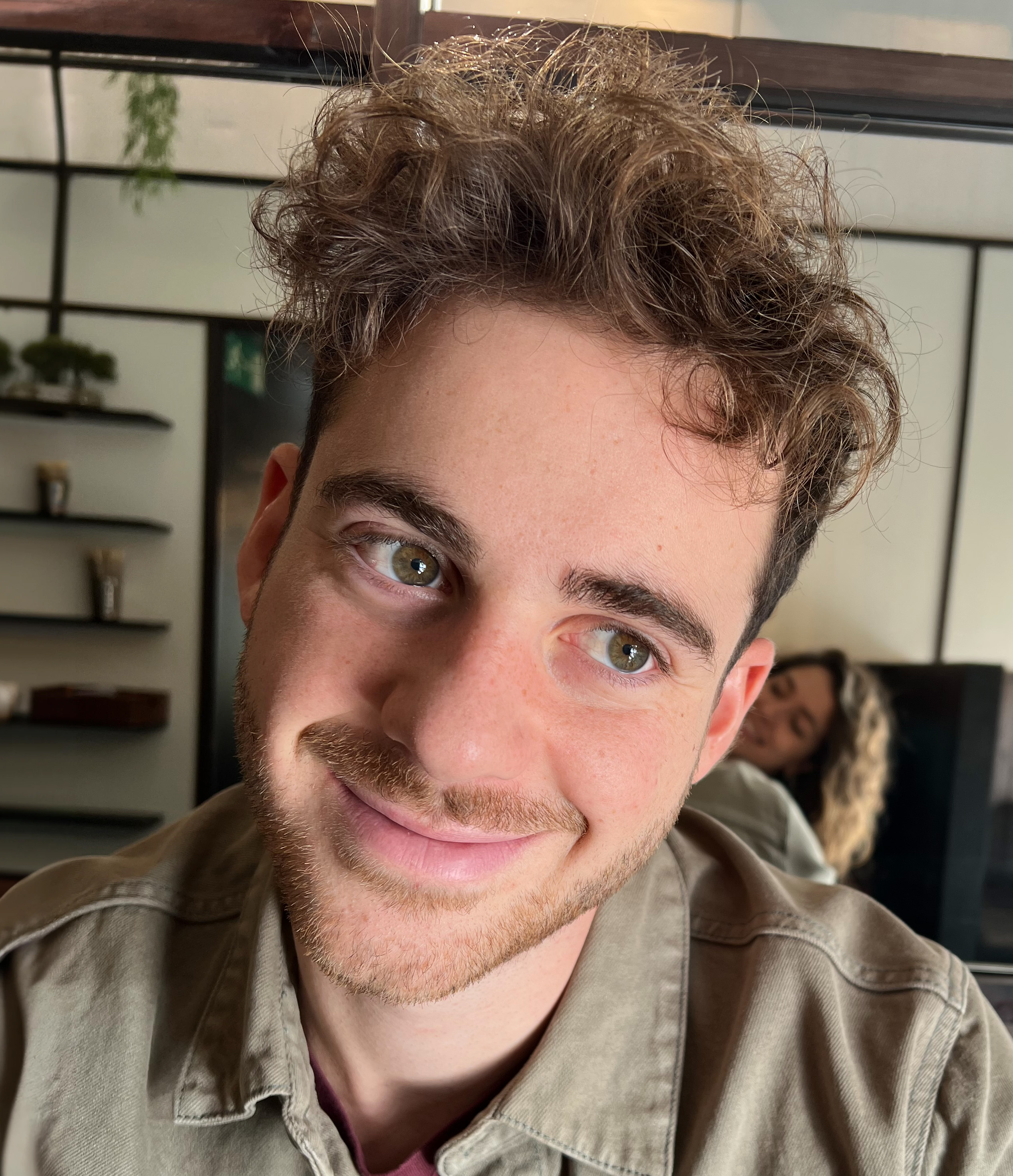
I am a final-year PhD student at Imperial College London, working on Graph Neural Networks and supervised by Prof. Michael Bronstein. Until November 2022, I also worked as a Machine Learning Researcher at Twitter. Prior to that, I was at Fabula AI, a startup developing a deep learning technology to detect fake news, which was then acquired by Twitter in June 2019. Previously, I completed an MPhil at the University of Cambridge and a BEng at Imperial College London, both in Computer Science.
My research focuses on bridging the gap between academic research and real-world applications of Graph Neural Networks. My co-authors I have developed methods for scaling Graph Neural Networks to large graphs, learning on temporal graphs, and dealing with missing node features. I prefer solutions that are simple to understand and implement, and are highly scalable. For example, see our SIGN model which is currently used in production at Airbnb to improve their trust & safety service predictions, or our TGN Model which has more than 750 Github stars. During my time at Twitter, I additionally worked on applied product problems such as the who-to-follow recommendation feature, for which I developed an embedding-based solution resulting in an increase of over 1.2M follow actions daily.
Away from machine learning, I recharge my batteries hiking, scuba diving, or just being immersed in nature.
News
Jul 31, 2023 | We are excited to release the Temporal Graph Benchmark, a collection of seven realistic, large-scale and diverse benchmarks for learning on temporal graphs! The accompanying paper has been accepted to NeurIPS 2023 Datasets and Benchmark track 📝 |
---|---|
Jun 8, 2023 | Our new paper on Graph Neural Networks for Directed Graphs, and how they improve learning on heterophilic graph, is out along with its associated blog post 📝 |
Apr 20, 2023 | New blog posts on our recent paper on Learning to Infer Structures of Network Games 📝 |
Jan 24, 2023 | Our paper “Graph Neural Networks for Link Prediction with Subgraph Sketching” has been accepted at ICLR 2022 as an oral presentation (top 5%) 🎉 |
Nov 24, 2022 | Our paper “On the Unreasonable Effectiveness of Feature propagation in Learning on Graphs with Missing Node Features” has been accepted at the new Learning on Graphs Conference 🎉 |